Why Sentiment Analysis Alone is Not Enough | Contextual AI for Reviews
Discover why sentiment analysis falls short in understanding reviews and how contextual AI powered by LLMs transforms customer insights.
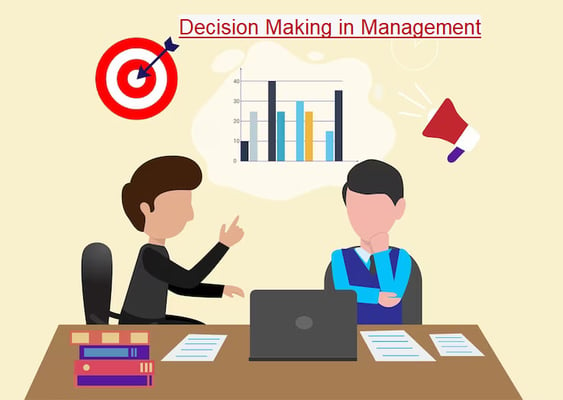
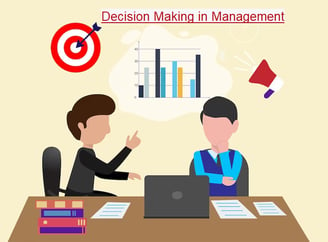
Customer reviews have become a cornerstone of decision-making for businesses and consumers alike. Whether it’s deciding on the best gadgets, skincare products, or healthcare services, reviews shape perceptions and drive purchases. While sentiment analysis has long been the go-to method for understanding customer feedback, it has its limitations. With advancements in Large Language Models (LLMs) like GPT-4, it’s time to explore the future of contextual AI in reviews.
The Limitations of Traditional Sentiment Analysis
Sentiment analysis, often categorized as positive, negative, or neutral, has served as the bedrock of customer feedback analysis. However, this approach is no longer sufficient to handle the complexities of modern consumer behavior.
1. Binary Classifications Lack Depth
Traditional sentiment analysis assigns a single polarity to reviews, failing to capture the nuances. For example, a review stating, "The product quality is amazing, but the customer service was disappointing," is inherently mixed. Sentiment analysis often misclassifies such feedback, leading to inaccurate insights.
2. Lack of Context Awareness
Sentiment analysis algorithms struggle to understand contextual nuances like sarcasm, idiomatic expressions, or domain-specific language. A sarcastic comment like, "Great! Another feature I didn’t ask for," could be flagged incorrectly as positive.
3. Focus on Words, Not Intent
Most sentiment models prioritize word-level analysis, ignoring broader contexts. For example, the word "cheap" could have a positive connotation in some industries (affordable pricing) and a negative one in others (low quality).
4. Fails to Address Customer Priorities
Sentiment analysis doesn’t differentiate between critical product features and minor grievances. For instance, a customer complaining about packaging versus one highlighting defective product functionality are not given proportional weight.
5. Static Rules in a Dynamic World
Consumer language evolves, introducing new phrases and slang. Static sentiment models are unable to keep up with these changes.
The Rise of Contextual AI with LLMs
Large Language Models, powered by deep learning, offer a transformative approach to customer review analysis. Unlike traditional sentiment analysis, LLMs are designed to understand context, extract themes, and provide a holistic view of customer sentiment.
How LLMs Add Depth to Review Analysis
Contextual Understanding
LLMs excel at understanding the intent behind words and phrases. They can distinguish between sarcasm, genuine praise, and constructive criticism, ensuring a more accurate interpretation of customer sentiment.
Topic Modeling
By grouping reviews into clusters of related topics, LLMs help businesses pinpoint specific areas of improvement or strengths. For instance, reviews on an e-commerce platform can be categorized into shipping experience, product quality, and customer service.
Sentiment Layering
Instead of a binary or ternary sentiment classification, LLMs provide a sentiment gradient. This allows businesses to understand the degree of positivity or negativity in customer feedback.
Customization for Industries
LLMs can be fine-tuned for specific industries. For example, healthcare reviews may focus on empathy and diagnosis accuracy, while tech product reviews highlight features and ease of use.
Predictive Analytics
With their ability to process large volumes of historical data, LLMs can predict customer behavior trends. This can guide marketing strategies, product enhancements, and customer engagement.
Real-World Applications of Contextual AI in Reviews
CustomerReviewMonitoring.com: The Next-Gen Solution
At CustomerReviewMonitoring.com, we’ve embraced LLM-powered contextual AI to revolutionize how businesses handle customer feedback.
Feature Analysis: Identify which product or service features resonate most with customers.
Customer Prioritization: Address critical issues first by categorizing reviews based on impact.
Real-Time Insights: Stay ahead with daily scraping and updates.
Scalable Solutions: Whether you manage 10 or 10,000 profiles, our platform is built to grow with your needs.
Breaking Down the Competitive Edge
Efficiency in Processing Large Data Volumes
Traditional sentiment tools crumble under the pressure of analyzing millions of reviews. LLMs, however, process massive datasets efficiently, uncovering hidden trends and actionable insights.
Enhanced Decision-Making
Businesses can use insights from LLMs to make data-driven decisions. Whether it’s tweaking a marketing campaign or redesigning a product feature, contextual AI ensures precision.
Personalization Opportunities
Contextual AI can extract individual customer preferences, enabling hyper-personalized marketing strategies and better customer retention.
Conclusion
While traditional sentiment analysis has its merits, it’s no longer sufficient to meet the demands of modern customer feedback analysis. The advent of LLM-powered contextual AI offers businesses a profound advantage by understanding the nuances of human language. By adopting advanced tools like those offered at CustomerReviewMonitoring.com, businesses can unlock the full potential of their customer reviews.
The future of review analysis lies in context, and with contextual AI, businesses can stay ahead in an increasingly competitive market.
Ready to Transform Your Review Insights?
Visit CustomerReviewMonitoring.com to learn how we can help you harness the power of contextual AI for unparalleled customer understanding.