What is Google AI Co-Scientist and How It Works?
Discover how Google AI Co-Scientist, powered by Gemini 2.0, uses multi-agent systems to generate novel research hypotheses and speed scientific breakthroughs.
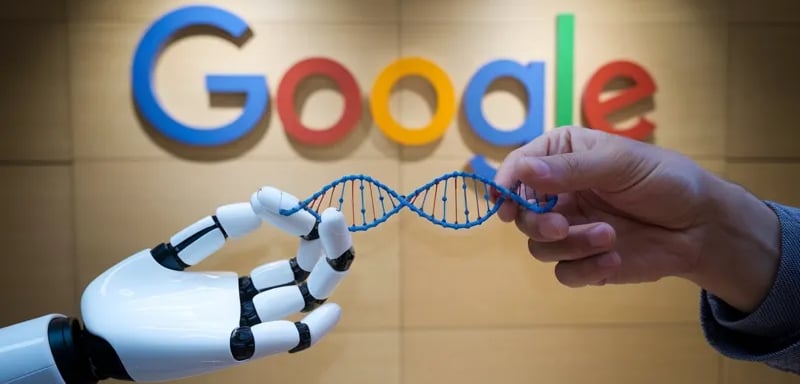
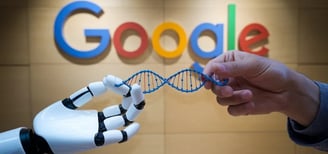
Google AI Co-Scientist is an innovative tool designed to address this challenge by augmenting the research process with advanced artificial intelligence. Built on the cutting-edge Gemini 2.0 platform, this multi-agent system serves as a collaborative partner for scientists, guiding them through the maze of modern research.
The Concept Behind AI Co-Scientist
At its core, Google AI Co-Scientist is modeled after the scientific method. It is not just a simple data retrieval tool—it is a system that:
Generates Novel Hypotheses: Proposes new research directions that might not emerge from traditional methods.
Refines Ideas: Iteratively improves upon its initial outputs through self-review and feedback.
Accelerates Discovery: Helps scientists quickly converge on promising experimental paths.
By combining computational power with a structured, methodical approach, the AI co-scientist acts as a virtual collaborator, enabling researchers to explore innovative ideas with greater efficiency and depth.
The Multi-Agent Framework
One of the standout features of the AI Co-Scientist is its multi-agent architecture. This framework consists of several specialized agents, each designed to mimic different aspects of the scientific process:
Generation Agent:
This agent kick-starts the process by generating initial hypotheses based on the research goals provided in natural language. It leverages vast databases of scientific literature to propose ideas that are both creative and grounded in existing knowledge.Reflection Agent:
After hypotheses are generated, the Reflection agent examines them closely, comparing new ideas with established research. This process ensures that the proposals are scientifically viable and relevant.Ranking Agent:
Not all ideas are created equal. The Ranking agent evaluates and orders hypotheses based on an automated quality metric, which helps in selecting the most promising directions for further exploration.Evolution Agent:
Science is an iterative process, and so is the AI co-scientist. The Evolution agent refines hypotheses over multiple cycles by integrating feedback and gradually enhancing the quality of the proposals.Proximity Agent:
This agent ensures that the generated ideas remain closely aligned with the specific research objectives. It acts as a guide, keeping the research focused and pertinent to the task at hand.Meta-Review Agent:
Overseeing the entire process, the Meta-Review agent performs a final evaluation. It integrates all insights from the specialized agents to deliver a comprehensive research overview, complete with detailed experimental protocols.
A central Supervisor agent coordinates these specialized agents. It dissects the research goal into manageable tasks and assigns them to the appropriate agents, ensuring smooth and effective collaboration.
Harnessing Test-Time Compute Scaling
A key innovation behind Google AI Co-Scientist is its use of test-time compute scaling. This advanced feature allows the system to:
Engage in Self-Play Debates: The system simulates scientific debates internally, using self-play techniques to compare and contrast different hypotheses.
Conduct Ranking Tournaments: By pitting multiple ideas against each other, the AI refines its proposals, continuously selecting and evolving the best candidates.
Iterate for Improvement: With each cycle of reasoning, the system leverages increased computational power to improve the quality and novelty of its outputs.
This iterative process is guided by an auto-evaluation metric derived from Elo ratings. Higher Elo ratings correlate with higher-quality hypotheses, ensuring that the system’s output meets rigorous scientific standards.
Real-World Applications and Validation
Google AI Co-Scientist isn’t just a theoretical tool—it has been tested and validated in real-world scenarios, particularly in biomedical research:
Drug Repurposing for Acute Myeloid Leukemia (AML)
In one compelling example, the AI co-scientist was tasked with identifying new therapeutic uses for existing drugs. For acute myeloid leukemia, it generated several candidate drugs that were then experimentally validated. These candidates demonstrated the ability to inhibit tumor viability at clinically relevant concentrations, showcasing the system’s potential to accelerate drug discovery.
Advancing Target Discovery in Liver Fibrosis
Another application focused on identifying novel targets for liver fibrosis treatment. The system successfully pinpointed epigenetic targets with strong anti-fibrotic potential. These results, validated in human hepatic organoid models, highlight how AI-assisted target discovery can streamline and enhance the efficiency of biomedical research.
Unraveling Mechanisms of Antimicrobial Resistance
The AI co-scientist also explored complex biological processes, such as the mechanisms behind antimicrobial resistance. By analyzing genetic data and existing literature, the system proposed novel explanations for bacterial gene transfer mechanisms. These insights were later supported by experimental data, further validating the approach.
Limitations and Future Directions
While Google AI Co-Scientist represents a significant leap forward, it is not without challenges. Current limitations include:
Enhanced Literature Review: There is ongoing work to improve the depth and accuracy of the literature review process.
Factuality Checking: Future iterations will incorporate more robust mechanisms to verify the factual accuracy of generated hypotheses.
Cross-Tool Integration: The system aims to integrate additional external tools to further enhance its evaluation and validation processes.
The research community is actively working to address these challenges, with the goal of creating an even more reliable and powerful tool for scientific discovery.
Conclusion
Google AI Co-Scientist exemplifies how artificial intelligence can augment human ingenuity and accelerate the pace of scientific breakthroughs. By harnessing a multi-agent framework and leveraging test-time compute scaling, this innovative system generates novel, testable hypotheses and refines them through iterative self-improvement. As it enters Trusted Tester programs worldwide, Google AI Co-Scientist is poised to transform the research landscape, opening new avenues for exploration in fields ranging from computer science to biomedicine.
In a world where rapid innovation is key, AI Co-Scientist stands as a promising testament to the future of collaborative, technology-enhanced research.
Explore Detail here https://research.google/blog/accelerating-scientific-breakthroughs-with-an-ai-co-scientist/