What is Chain-of-Thought Prompting Techniques?
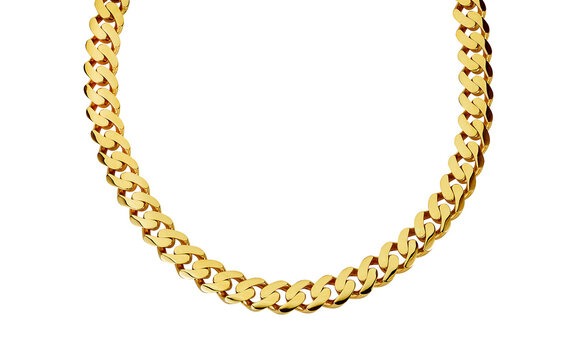
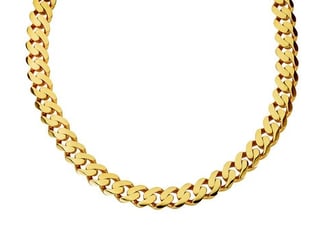
Artificial intelligence (AI), innovations continue to push the boundaries of what machines can achieve. Chain-of-thought prompting (COT) emerges as a significant advancement, aimed at enhancing AI systems' ability to reason and make decisions in a manner that mirrors human cognition.
What is Chain-of-Thought Prompting?
Chain-of-thought prompting (COT) is a technique within AI that involves generating prompts sequentially, where each subsequent prompt builds upon the context established by the previous one. Unlike traditional single-prompt approaches, COT allows AI models to simulate multi-step reasoning processes, thereby enabling more nuanced decision-making and complex problem-solving capabilities.
Traditional Prompting Techniques
To understand the significance of COT, it's essential to contrast it with conventional prompt-based models. While traditional prompts provide single inputs to AI systems, COT introduces a dynamic sequence of prompts that mimic the logical flow of human thoughts. This evolution is pivotal in advancing AI towards more sophisticated forms of intelligence.
Techniques and Implementations of Chain-of-Thought Prompting
Overview of COT Techniques
COT techniques encompass a spectrum of methodologies designed to enhance context awareness and decision-making in AI. These techniques include:
Chaining Prompts: Sequential generation of prompts where each subsequent prompt is influenced by the output of the previous one.
Recursive Prompting: Iterative refinement of prompts based on feedback loops, allowing AI systems to iteratively improve their understanding of complex tasks.
Multimodal Integration: Incorporation of diverse data types (text, images, audio) into prompt sequences to enrich context and enhance comprehension.
Real-World Applications
provides insights into practical applications of COT across industries:
Healthcare: Enhancing diagnostic accuracy by synthesizing patient data across multiple prompts, facilitating personalized treatment plans and medical decision support systems.
Finance: Improving fraud detection and risk assessment through interconnected prompts that analyze transactional data for anomalies and patterns indicative of fraudulent activity.
Technical Insights and Optimization Strategies
Parallel Processing: Distributing computational tasks across multiple processors to enhance efficiency and scalability.
Adaptive Learning Algorithms: Iterative adjustments to model parameters based on real-time data inputs, improving adaptability and performance in dynamic environments.
Understanding Human Reasoning through Chain-of-Thought Prompting
Inference and Abstraction: How COT enables AI systems to infer implicit information and abstract complex concepts from sequential prompts, akin to human reasoning.
Contextual Understanding: The role of context in shaping decision-making processes within COT frameworks, highlighting the importance of context-aware AI models in achieving human-like intelligence.
Applications Across Industries
Healthcare
Speech Recognition: Improving accuracy in transcribing medical conversations by chaining prompts that capture nuanced linguistic and contextual cues.
Clinical Decision Support: Assisting healthcare providers in diagnosing and treating patients by integrating multi-dimensional prompt sequences that consider medical histories, symptoms, and diagnostic test results.
Finance
Predictive Analytics: Forecasting market trends and customer behavior patterns through iterative prompt sequences that analyze historical data and real-time market indicators.
Risk Management: Identifying potential risks and optimizing investment strategies by leveraging interconnected prompts to evaluate financial transactions and market fluctuations.
Advantages and Challenges of Chain-of-Thought Prompting
Benefits
Enhanced Context Awareness: Ability to consider multiple dimensions of information and interrelationships between data points, leading to more informed decision-making.
Complex Problem-Solving: Facilitation of intricate problem-solving tasks that require sequential reasoning and integration of diverse data sources.
Adaptability Across Domains: Applicability of COT techniques in diverse industries, from healthcare and finance to education and cybersecurity.
Challenges
Training Complexity: The intricacies involved in training COT models due to sequential dependencies and the need for iterative learning processes.
Scalability Issues: Challenges in scaling COT frameworks to handle large volumes of data and real-time processing requirements in enterprise-scale applications.
Ethical Considerations: Addressing privacy concerns, algorithmic bias, and transparency in deploying COT-enabled systems in sensitive domains.
Future Trends and Research Directions in Chain-of-Thought Prompting
Emerging Technologies
Future advancements in COT are poised to:
Integrate Multimodal Inputs: Enhance AI systems' capability to process and interpret diverse data types (text, image, audio) within unified prompt sequences.
Improve Model Interpretability: Develop hybrid models that combine COT with explainable AI techniques to enhance transparency and interpretability in decision-making processes.
Research Initiatives
Ongoing research initiatives focus on:
Algorithmic Innovation: Developing novel algorithms and architectures that optimize COT performance in dynamic and evolving environments.
Human-AI Collaboration: Exploring synergies between human cognition and COT frameworks to augment human decision-making capabilities across various domains.
Ethical Considerations in Deploying Chain-of-Thought Prompting
Ensuring ethical deployment of COT involves:
Data Privacy: Implementing robust data governance frameworks to protect sensitive information used in prompt sequences.
Bias Mitigation: Addressing algorithmic biases that may arise from skewed training data or inadequate representation of diverse demographic groups.
Conclusion
Chain-of-thought prompting represents a paradigm shift in AI, moving towards more human-like reasoning and decision-making capabilities. As advancements continue to unfold, COT holds immense potential to transform industries, enhance societal well-being, and redefine the boundaries of artificial intelligence.