Top 10 LLM Interview Questions 📚🤖
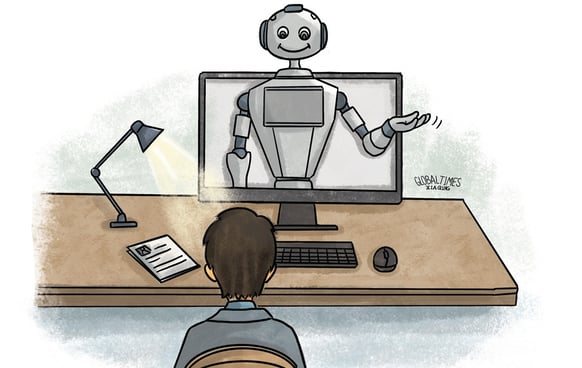
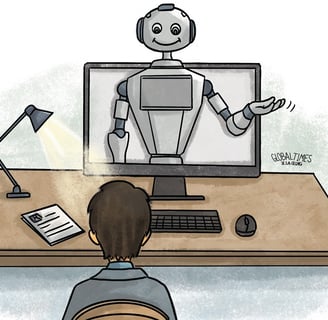
1.What is LLM?
Ans: large language model (LLM) is a smart computer program that can understand and create human-like text. It learns this by studying vast amounts of data from sources like the internet, where it reads millions of pages to see how words and sentences are used. This type of learning is called machine learning, specifically using a method called deep learning.
The model is based on a neural network called a transformer, which helps it process and understand language patterns. After being trained on all this data, the model can then be fine-tuned to specialize in different tasks, like answering questions or translating languages. This makes LLMs incredibly useful for tasks that involve understanding and generating written or spoken language, without needing constant human input.
2.What is a Transformer? Describe the Transformer architecture and its role in building Large Language Model (LLM) applications?
Ans: A Transformer is a type of neural network architecture introduced in the paper "Attention Is All You Need" by Vaswani et al. (2017). It has become foundational in natural language processing (NLP) and the development of large language models (LLMs).
Transformer Architecture
The Transformer architecture consists of an encoder and a decoder, each composed of a stack of identical layers. Here's a breakdown of its components:
Encoder: The encoder processes the input sequence and outputs a representation of it.
Self-Attention Mechanism: Allows the model to focus on different parts of the input sequence when encoding a particular token.
Feed-Forward Neural Network: Applied to each position separately and identically.
Layer Normalization and Residual Connections: Improve training and convergence.
Decoder: The decoder generates the output sequence, using the encoder's output and the previously generated tokens.
Masked Self-Attention: Ensures that each position in the decoder can only attend to earlier positions in the output sequence.
Encoder-Decoder Attention: Enables the decoder to focus on relevant parts of the input sequence.
Positional Encoding: Since the Transformer lacks an inherent sense of the order of the sequence, positional encodings are added to input embeddings to provide this information.
Role in Building LLM Applications
Transformers play a crucial role in building large language models like GPT-3, BERT, and others. Key aspects include:
Scalability: Transformers scale well with increased data and computational power, enabling the creation of very large models.
Parallelization: Unlike RNNs, Transformers allow for parallel processing of sequences, leading to faster training times.
Pre-training and Fine-tuning: Transformers can be pre-trained on vast amounts of text data and fine-tuned on specific tasks, improving performance on a wide range of NLP applications.
Comparison with RNNs, LSTMs, and BiLSTMs
Before Transformers, Recurrent Neural Networks (RNNs), Long Short-Term Memory networks (LSTMs), and Bidirectional LSTMs (BiLSTMs) were the primary architectures for sequence modeling tasks in NLP.
RNNs (Recurrent Neural Networks):
Process sequences one step at a time, maintaining a hidden state that carries information across steps.
Suffer from the vanishing gradient problem, making it difficult to learn long-term dependencies.
LSTMs (Long Short-Term Memory networks):
Address the vanishing gradient problem with a gating mechanism that regulates the flow of information.
Capable of learning long-term dependencies better than standard RNNs.
BiLSTMs (Bidirectional LSTMs):
Process the input sequence in both forward and backward directions, capturing context from both past and future states.
Enhance the model's ability to understand the context but still face limitations in parallelization.
3.Why Transformers Over RNNs, LSTMs, and BiLSTMs?
Efficiency and Parallelization: Transformers allow for parallel processing of all tokens in a sequence, significantly speeding up training and inference times compared to the sequential nature of RNNs and LSTMs.
Handling Long-Term Dependencies: The self-attention mechanism in Transformers enables them to capture long-range dependencies more effectively than RNNs, LSTMs, and BiLSTMs, which can struggle with very long sequences.
Scalability: Transformers scale better with data and model size, making them suitable for building very large language models like GPT-3 and BERT.
Versatility: The Transformer architecture is highly versatile and has been adapted for a wide range of tasks beyond language modeling, such as image processing and reinforcement learning.
Overall, the Transformer architecture has revolutionized NLP by enabling models that understand and generate human language with high accuracy and fluency, surpassing the capabilities of previous architectures like RNNs, LSTMs, and BiLSTMs.
4.Explain fine-tuning Process and how it can be used to customize pre-trained models for a specific task?
Fine-tuning is a process in machine learning where a pre-trained model is further trained on a specific dataset to adapt it to a particular task. This approach leverages the knowledge the model has already gained from a large, general-purpose dataset and refines it for more specialized tasks. Here’s a step-by-step explanation of fine-tuning and its application:
1. Pre-trained Models
Pre-trained models are neural networks that have been previously trained on large, diverse datasets (such as ImageNet for image models or large corpora for language models). These models have learned to extract general features and patterns from data.
2. Why Fine-tuning?
Training a neural network from scratch requires a lot of data and computational resources. Fine-tuning is efficient because it builds on the existing knowledge of the pre-trained model, requiring less data and computation. Fine-tuning is particularly useful when:
The target task has a smaller dataset.
The target task is similar but not identical to the pre-training task.
Computational resources are limited.
3. Fine-tuning Process
The fine-tuning process typically involves the following steps:
a. Load the Pre-trained Model
Start with a model that has been pre-trained on a large dataset. This model already knows how to extract useful features from data.
b. Modify the Model
Adjust the model architecture if necessary. Common modifications include:
Changing the output layer to match the number of classes in the new task (for classification problems).
Adding task-specific layers.
c. Freeze Some Layers
Freeze the weights of some of the earlier layers in the network. These layers usually contain general features that are useful for many tasks. Freezing them prevents their weights from being updated during fine-tuning, reducing the risk of overfitting and speeding up training.
d. Train on the New Dataset
Train the modified model on the new, task-specific dataset. Use a smaller learning rate to make fine adjustments to the weights. The model will learn to adapt the features it already knows to the specifics of the new task.
4. Benefits of Fine-tuning
Efficiency: Reduces the amount of data and computational power required compared to training from scratch.
Improved Performance: Often leads to better performance on the specific task because the model starts with a good understanding of general features.
Faster Development: Accelerates the development process as the base model has already learned a lot from the pre-training phase.
5. Example Applications
Image Classification: Using a model pre-trained on ImageNet to classify medical images, satellite images, or any specific category not covered in the general dataset.
Natural Language Processing (NLP): Fine-tuning models like BERT, GPT, or T5 on specific tasks such as sentiment analysis, named entity recognition, or custom text generation.
Speech Recognition: Adapting a general speech recognition model to recognize industry-specific jargon or different accents.
Fine-tuning is a powerful technique in transfer learning that allows leveraging pre-trained models for specialized tasks, saving time, resources, and often achieving superior performance compared to training models from scratch. It combines the broad knowledge of pre-trained models with the specificity of task-focused data, resulting in robust and efficient machine learning solutions.
5.Different between Training and fine-tuning?
Training and fine-tuning a Large Language Model (LLM) are fundamentally different processes, each with distinct goals and methodologies.
Training an LLM involves creating a model from scratch using a vast corpus of text data. This phase is extensive and resource-intensive, requiring significant computational power and large datasets. The goal is to enable the model to learn the nuances of human language, including grammar, context, facts, and even some reasoning abilities. During this process, the model adjusts its numerous parameters (often in the billions) through a process called self-supervised learning. This allows the model to generate coherent and contextually appropriate text based on the patterns it has learned from the training data.
Fine-tuning an LLM, on the other hand, is a more specialized process. It starts with an already pre-trained model and further trains it on a smaller, task-specific dataset. The purpose of fine-tuning is to adapt the general language model to perform well on a particular task, such as sentiment analysis, machine translation, or question-answering. Fine-tuning requires significantly less data and computational resources compared to the initial training phase. It typically involves adjusting a subset of the model’s parameters while retaining the foundational knowledge acquired during the initial training.
In summary, the key differences are:
Scope and Data: Training uses a broad and diverse dataset to teach the model general language skills, while fine-tuning uses a narrow, task-specific dataset to specialize the model.
Resource Intensity: Training is resource-intensive and time-consuming, whereas fine-tuning is comparatively quicker and less resource-demanding.
Purpose: Training builds the foundational capabilities of the model, while fine-tuning hones these capabilities for specific applications.
These processes together allow LLMs to be both broadly knowledgeable and highly specialized, depending on the needs of the application.
6.Explain Retrieval Augmented Generation (RAG) vs. Fine-Tuning?
1. Knowledge Integration vs. Task Specialization
RAG: Enhances model output by integrating external data sources in real-time, enabling context-aware responses without altering the model's inherent functioning. This makes RAG suitable for tasks requiring up-to-date or extensive information beyond the model's initial training set.
Fine-Tuning: Adapts a general-purpose model to specialize in a particular task by adjusting its internal parameters. This method refines the model’s ability to handle specific contexts, making it more effective for specialized tasks like legal document analysis or sentiment detection.
2. Dynamic vs. Static Learning
RAG: Employs a dynamic learning approach, allowing the model to access the latest information by querying updated databases or documents during inference. This keeps the model's responses current without the need for retraining.
Fine-Tuning: Involves static learning where the model's knowledge is fixed based on the dataset used during the tuning phase. The model may become outdated as new data emerges, necessitating periodic retraining to stay relevant.
3. Generalization vs. Customization
RAG: Excels in generalization by using retrieval mechanisms to adapt responses based on a wide range of accessible information. This makes it versatile for applications needing comprehensive knowledge.
Fine-Tuning: Focuses on customization, optimizing the model for specific scenarios closely aligned with the training data. This results in high precision and relevance for targeted applications but limits general versatility.
4. Resource Intensity
RAG: Requires significant computational power and memory during inference, leading to higher operational costs, especially when scaling for widespread use. However, it reduces the need for frequent model retraining.
Fine-Tuning: Computationally intensive during the tuning phase but efficient in serving user queries post-tuning. It avoids the continuous resource demands associated with RAG's real-time retrieval.
Use Cases
RAG: Suitable for applications like chatbots, legal research, and translation tasks where accessing the latest information or specialized content is crucial. RAG enhances the model’s ability to provide accurate and contextually relevant responses by integrating real-time data.
Fine-Tuning: Ideal for scenarios with stable, high-quality labeled datasets, such as personalized education, financial analysis, and sentiment analysis. It tailors the model to perform specific tasks with high precision, making it effective for specialized applications.
Choosing Between RAG and Fine-Tuning
The choice between RAG and fine-tuning depends on factors like the availability of domain-specific labeled data, the dynamic nature of the data, and the need for model transparency. RAG is advantageous in dynamic environments and where transparency is crucial, while fine-tuning excels in scenarios with abundant labeled data and a need for task-specific customization.
By understanding these distinctions, organizations can make informed decisions on which approach best suits their needs for enhancing LLM performance.
7.What is Transfer Learning? Importance of Transfer Learning for Large Language Models (LLMs)?
Transfer learning is a machine learning technique where a model developed for one task is reused as the starting point for a model on a second task. It is particularly useful when the second task has limited data. Instead of starting from scratch, transfer learning leverages the knowledge gained from solving one problem and applies it to a different but related problem
Transfer learning is critically important for large language models (LLMs) due to several key advantages it provides, enhancing both the efficiency and effectiveness of these models in various applications.
Reduced Training Time and Resources
Transfer learning significantly cuts down the time and computational resources required to train LLMs. Instead of training a model from scratch, which demands vast amounts of data and computational power, transfer learning leverages pre-trained models that have already learned general language features. These pre-trained models can then be fine-tuned on smaller, task-specific datasets, which is much less resource-intensive
Improved Performance
Pre-trained models used in transfer learning have already captured a wealth of linguistic patterns and knowledge from large and diverse datasets. This foundational understanding allows them to perform better when fine-tuned for specific tasks, such as sentiment analysis, named entity recognition, text classification, and question answering. By building on this pre-existing knowledge, fine-tuned models tend to achieve higher accuracy and more reliable outputs compared to models trained from scratch
Versatility Across Domains
Transfer learning enhances the versatility of LLMs by enabling them to be adapted to a wide range of domains with relatively little additional training. For example, models like GPT-4, BERT, and T5 can be fine-tuned for specialized tasks in diverse fields such as healthcare, finance, and customer service. This adaptability is crucial for applying LLMs to specific industry needs without the prohibitive cost of training new models for each unique application.
Addressing Data Scarcity
In many specialized fields, labeled training data is scarce. Transfer learning mitigates this issue by allowing models to be fine-tuned on small datasets while still achieving high performance. This is particularly beneficial for tasks where gathering large amounts of labeled data is impractical or costly (Spot Intelligence).
Ongoing Adaptation and Improvement
Transfer learning facilitates continuous improvement of LLMs. As new data becomes available or as the specific requirements of tasks evolve, pre-trained models can be incrementally fine-tuned to adapt to these changes. This dynamic adaptability ensures that models remain relevant and effective over time (
Practical Applications
The practical applications of transfer learning in LLMs are vast. In marketing, it can be used to generate personalized content; in healthcare, it helps in organizing patient data and improving diagnostics; in education, it assists in creating customized learning experiences. These applications demonstrate how transfer learning extends the utility of LLMs beyond general language tasks to specific, high-impact areas
In summary, transfer learning is a transformative approach for leveraging large language models, providing significant benefits in terms of efficiency, performance, adaptability, and practical application across various domains.
8.Limitations large language models (LLMs) ?
large language models (LLMs) comes with several challenges and limitations, despite their impressive capabilities. Here are some of the key issues:
Bias and Fairness: LLMs are trained on vast datasets that may contain biased information, leading the models to inadvertently learn and reproduce these biases. This can result in biased outputs that may perpetuate stereotypes or unfair treatment of certain groups (Stanford NLP) (O'Reilly Media).
Resource Intensity: Training and operating LLMs require substantial computational resources, including powerful hardware and significant energy consumption. This makes them expensive to develop and maintain, and raises concerns about their environmental impact (O'Reilly Media).
Interpretability and Transparency: LLMs function as "black boxes," meaning their decision-making processes are not easily understood even by their developers. This lack of interpretability makes it challenging to debug models, ensure accountability, and trust their outputs in critical applications (O'Reilly Media).
Contextual Understanding and Reasoning: While LLMs are excellent at generating human-like text, they often lack true understanding and reasoning capabilities. They can produce coherent responses without genuinely comprehending the context, which can lead to plausible but incorrect or nonsensical answers (Stanford NLP) (O'Reilly Media).
Safety and Ethical Concerns: LLMs can be misused for harmful purposes, such as generating fake news, deepfakes, or other malicious content. Ensuring the ethical use of these models and preventing misuse is a significant ongoing challenge (O'Reilly Media).
Data Privacy: The data used to train LLMs can sometimes include sensitive information. Ensuring that models do not inadvertently leak personal data or confidential information is crucial for maintaining privacy standards (Stanford NLP).
Addressing these challenges requires ongoing research, improved training methodologies, better interpretability tools, and robust ethical guidelines to ensure that LLMs are developed and used responsibly.
9.Explain Attention Mechanisms in Large Language Models (LLMs)?
Ans: Attention mechanisms are a fundamental component of large language models (LLMs) like GPT-3 and BERT, allowing them to process and understand language effectively. Here’s a detailed overview of how these mechanisms work and why they are so powerful:
Core Concepts of Attention Mechanisms
Self-Attention: Self-attention, or intra-attention, is a process where each word in a sentence is related to every other word. It helps the model focus on relevant parts of the input sentence when producing a representation for each word. This is done using three vectors: Query, Key, and Value.
Query, Key, and Value:
Query (Q): Represents the word for which the model is trying to find relevant context.
Key (K): Represents potential words that might match with the query.
Value (V): Contains the actual information of the word, which is weighted and summed up based on the relevance determined by the key-query pair.
The attention mechanism calculates a score by comparing the query vector with key vectors of other words. These scores determine how much focus each word should receive, creating a weighted sum of the value vectors.
Multi-Head Attention: This involves running multiple self-attention operations in parallel, each with different projections of Q, K, and V. This allows the model to capture various aspects of the relationships between words, improving its understanding and contextual representation.
How Attention Mechanisms Work
Weighted Sum: The core idea of attention is to compute a weighted sum of the value vectors. The weights are derived from the compatibility function that measures the similarity between the query and key vectors.
Compatibility Function: A common approach is to use dot-product attention, where the dot product of the query and key vectors is taken, often followed by scaling and applying a softmax function to get the weights.
Focus and Context: In a sentence, some words are more important than others for understanding the context. Attention mechanisms enable the model to dynamically focus on relevant words while processing input data, enhancing its ability to understand and generate language.
Benefits of Attention Mechanisms
Parallel Processing: Unlike Recurrent Neural Networks (RNNs), which process sequences sequentially, attention mechanisms allow LLMs to process all words in a sequence simultaneously. This results in faster training times and better handling of long-range dependencies.
Handling Long-Range Dependencies: Self-attention enables models to relate distant words in a sentence, which is crucial for understanding context over long text spans, such as in machine translation or summarization tasks.
Scalability: Attention mechanisms scale efficiently, making them suitable for large-scale models with billions of parameters like GPT-3.
Challenges and Refinements
While powerful, attention mechanisms also present challenges such as computational inefficiency and potential biases from training data. Ongoing research aims to optimize these mechanisms and address biases to make models more fair and transparent.
In summary, attention mechanisms are integral to the functioning of LLMs, providing the ability to focus on relevant parts of the input data, capture long-range dependencies, and process information in parallel. These capabilities are foundational to the impressive performance of models like GPT-3 and BERT.
10.What is Hallucination in Large Language Models (LLMs) and How Can it Be Prevented?
Ans: Understanding Hallucinations in LLMs
Hallucination in the context of large language models (LLMs) refers to instances where the model generates text that is factually incorrect, nonsensical, or not grounded in the provided context. This can occur due to various reasons, including flaws in training data, model architecture, or inference processes.
Causes of Hallucinations
Training Data Issues: LLMs are trained on vast and diverse datasets sourced from the internet, which may include inaccurate or biased information. This can lead to models generating outputs that replicate these inaccuracies (Lakera).
Model Architecture and Training Objectives: Structural flaws in the model or misaligned training objectives can result in the generation of incorrect outputs
Inference Stage Challenges: During the text generation process, randomness in sampling methods and insufficient attention to context can lead to hallucinations
Prompt Engineering: Ambiguously worded or context-lacking prompts can cause models to produce irrelevant or incorrect responses.
Semantic Gaps: LLMs may lack common sense reasoning and real-world experience, contributing to hallucinations.
Detecting Hallucinations
Fact Verification: Cross-referencing generated information with reliable sources to check for accuracy (Simform).
Contextual Understanding: Analyzing if the generated text aligns with the query or conversation history.
Adversarial Testing: Creating challenging prompts to expose hallucination patterns
Consistency Analysis: Checking for logical consistency within the text.
Chain of Thought Prompting: Asking the model to explain its reasoning step-by-step to identify logical gaps.
Preventing Hallucinations
Curated Datasets: Using high-quality, verified datasets for training can reduce the likelihood of hallucinations.
Output Filtering: Implementing mechanisms to flag or filter potentially incorrect outputs based on statistical likelihood or domain-specific rules.
Feedback Mechanism: Establishing a real-time user feedback system to fine-tune the model continuously based on user inputs.
Iterative Fine-Tuning: Regularly updating the model with more accurate and recent datasets.
Cross-Referencing: Verifying critical outputs with trusted information sources .
Domain-Specific Training: Involving experts to fine-tune models for specific fields like healthcare or law to reduce inaccuracies (Iguazio).
Monitoring and MLOps: Continuous monitoring of the model's performance, including automated data validation and feedback loops, can help detect and correct hallucinations.
Ethical Concerns
Hallucinations in LLMs can lead to the spread of misinformation, impaired judgment, loss of trust in AI technologies, and reinforcement of biases present in the training data. Therefore, it is crucial to address and mitigate these risks to ensure the reliability and ethical use of AI systems (Iguazio).
By understanding the causes and implementing these preventive measures, the occurrence of hallucinations in LLMs can be significantly reduced, improving the accuracy and trustworthiness of AI-generated content.