The Next Platform for Brain-Inspired Computing
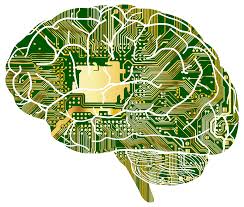
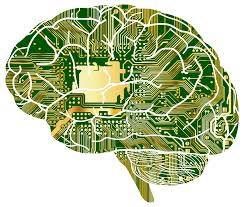
Computers have made incredible strides in power and potential, surpassing human brains in tasks like data storage, crunching numbers, making predictions, and communication. Yet, one domain where human brains still hold a significant edge is energy efficiency. This distinction is crucial as the world grapples with growing energy consumption driven by the rapid proliferation of on-chip electronics.
"The most efficient computers are still approximately four orders of magnitude — that's 10,000 times — higher in energy requirements compared to the human brain for specific tasks such as image processing and recognition, although they outperform the brain in tasks like mathematical calculations," says Professor Kaustav Banerjee from UC Santa Barbara, an expert in nanoelectronics. "Making computers more energy efficient is crucial because the worldwide energy consumption by on-chip electronics stands at #4 in the global rankings of nation-wise energy consumption, and it is increasing exponentially each year, fueled by applications such as artificial intelligence" Furthermore, the issue of energy inefficiency in computing is particularly urgent in the context of global warming, underscoring the need to develop more energy-efficient technologies.
Neuromorphic Computing
Neuromorphic (NM) computing has emerged as a promising approach to narrowing the energy efficiency gap between human brains and computers. By mimicking the structure and operations of the human brain, where processing occurs in parallel across an array of low-power-consuming neurons, neuromorphic computing holds the potential to achieve brain-like energy efficiency. In a groundbreaking paper published in Nature Communications, Banerjee and his colleagues — Arnab Pal, Zichun Chai, Junkai Jiang, Wei Cao, Vivek De, and Mike Davies from Intel Labs — propose an ultra-energy-efficient platform using 2D transition metal dichalcogenide (TMD)-based tunnel-field-effect transistors (TFETs). Their platform could reduce energy requirements to within two orders of magnitude (about 100 times) compared to the human brain.
The Promise of 2D Tunnel-Transistors
The concept of neuromorphic computing has been around for decades, but research in this area has intensified only recently. Advances in circuitry that enable smaller, denser arrays of transistors — and therefore more processing and functionality for less power consumption — are just beginning to unlock the potential of brain-inspired computing. The growing demand for applications such as AI and the Internet-of-Things further highlights the need to develop new hardware platforms for neuromorphic computing.
Banerjee's team has developed 2D tunnel-transistors, emerging from his extensive research efforts to create high-performance, low-power transistors that address the increasing demand for processing power without a corresponding rise in energy consumption. These atomically thin, nanoscale transistors are highly responsive at low voltages. As the foundation of the researchers' NM platform, they can mimic the human brain's highly energy-efficient operations. In addition to having lower off-state currents, the 2D TFETs boast a low subthreshold swing (SS), a parameter that measures a transistor's ability to switch from off to on. A lower SS translates to a lower operating voltage and faster, more efficient switching.
"Neuromorphic computing architectures are designed to operate with very sparse firing circuits," explains lead author Arnab Pal. "This means they mimic how neurons in the brain fire only when necessary." In contrast to the conventional von Neumann architecture of today's computers — where data is processed sequentially, and memory and processing components are separated and continuously draw power throughout the operation — an event-driven NM computer fires up only when there is input to process. Memory and processing are distributed across an array of transistors. Companies like Intel and IBM have already developed brain-inspired platforms, deploying billions of interconnected transistors and achieving significant energy savings.
Overcoming the Challenge of Leakage Currents
Despite these advances, there is still room for improvement in energy efficiency. "In these systems, most of the energy is lost through leakage currents when the transistors are off, rather than during their active state," Banerjee explains. Leakage currents are small amounts of electricity that flow through a circuit even when it is off but still connected to power. Current NM chips use traditional metal-oxide-semiconductor field-effect transistors (MOSFETs), which have high on-state current but also high off-state leakage. "Since the power efficiency of these chips is constrained by the off-state leakage, our approach — using tunneling transistors with much lower off-state current — can greatly improve power efficiency," Banerjee adds.
When integrated into a neuromorphic circuit that emulates the firing and reset of neurons, TFETs have proven to be more energy-efficient than state-of-the-art MOSFETs, particularly FinFETs (a MOSFET design that incorporates vertical "fins" to provide better control of switching and leakage). Although TFETs are still in the experimental stage, their performance and energy efficiency make them a promising candidate for the next generation of brain-inspired computing.
Looking Ahead
According to co-authors Vivek De and Mike Davies from Intel, "Once realized, this platform can bring the energy consumption in chips to within two orders of magnitude with respect to the human brain — not accounting for the interface circuitry and memory storage elements. This represents a significant improvement from what is achievable today."
Banerjee envisions that future iterations of these 2D-TFET-based neuromorphic circuits could take the form of three-dimensional structures, offering even closer emulation of the human brain. Widely recognized as one of the key visionaries behind 3D integrated circuits now witnessing wide-scale commercial adoption, Banerjee sees this as the next frontier in the quest for energy-efficient computing.
The journey towards achieving brain-like energy efficiency in computing is a testament to the ingenuity and perseverance of researchers and engineers. As we stand on the brink of this new era, the promise of neuromorphic computing holds the potential to revolutionize the way we think about and use technology, paving the way for a more sustainable and energy-efficient future.