Innovative Training Technique Aims to Reduce Social Bias in AI Systems
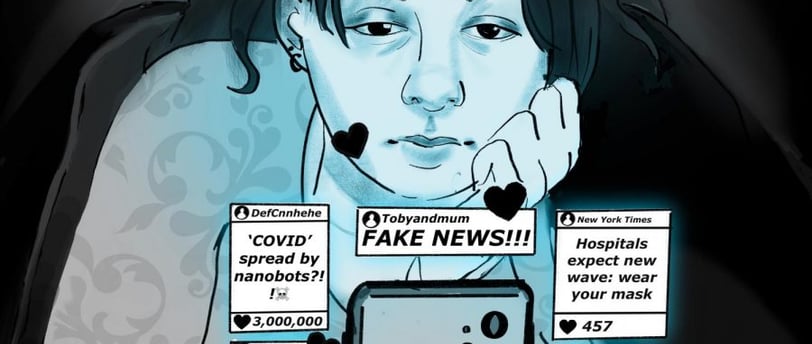
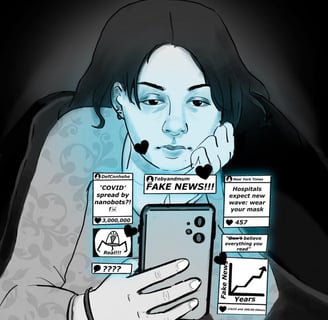
Artificial Intelligence (AI) has become a cornerstone of modern technology, driving advancements across various industries. However, as AI systems evolve, concerns about their inherent biases have intensified. These biases often stem from the datasets used to train AI, which can inadvertently perpetuate social prejudices present in society. In a groundbreaking development, a team of researchers from Oregon State University and Adobe has introduced a novel training technique designed to mitigate these biases, making AI systems more equitable and fair.
The Need for Fair AI
AI systems learn from vast amounts of data, and these datasets frequently reflect the biases existing in human societies. When AI models are trained on biased data, they can perpetuate and even exacerbate these biases, leading to unfair and discriminatory outcomes. For instance, an AI system might disproportionately display images of white men when asked to show pictures of CEOs or doctors, reinforcing gender and racial stereotypes.
Addressing these biases is crucial for the development of socially just AI systems. Researchers are actively seeking methods to reduce biases in AI training processes to ensure that AI technologies benefit all individuals fairly.
Introducing FairDeDup
The new technique, named FairDeDup, was developed by Eric Slyman, a doctoral student at Oregon State University's College of Engineering, in collaboration with researchers Scott Cohen and Kushal Kafle from Adobe. FairDeDup stands for fair deduplication, a process that involves removing redundant information from datasets used for AI training. By doing so, it aims to reduce the computational costs associated with AI training while simultaneously addressing social biases.
How FairDeDup Works
FairDeDup builds upon an earlier method known as SemDeDup, which focused on cost-effective AI training through deduplication. While SemDeDup successfully reduced training costs, it inadvertently exacerbated social biases in AI models. FairDeDup improves upon this by incorporating fairness considerations into the deduplication process.
The technique involves a process called pruning, where a representative subset of data is selected from a larger dataset. By making content-aware decisions during pruning, FairDeDup ensures that the selected subset is diverse and representative, thus reducing biases in the training data.
Addressing Multiple Bias Dimensions
FairDeDup targets various dimensions of bias, including race, gender, occupation, age, geography, and culture. By understanding how deduplication affects these biases, the technique mitigates their prevalence in AI models. This approach enables the creation of AI systems that are not only cost-effective and accurate but also more socially just.
The Impact of FairDeDup
The introduction of FairDeDup represents a significant step forward in the quest for fair AI. By reducing biases in training datasets, this technique can help create AI systems that are more inclusive and equitable. The impact of FairDeDup extends beyond just technical improvements; it has profound implications for how AI technologies are developed and deployed in society.
Real-World Applications
The potential applications of FairDeDup are vast and varied. Here are a few examples of how this technique can be applied in different domains:
Healthcare: AI systems used in healthcare can benefit from FairDeDup by ensuring that diagnostic tools and treatment recommendations are fair and unbiased, leading to better patient outcomes across diverse populations.
Hiring and Recruitment: In the hiring process, AI tools can be used to screen candidates fairly, reducing biases related to gender, race, and other factors.
Education: FairDeDup can help create educational AI systems that provide equal learning opportunities to students from all backgrounds.
Law Enforcement: AI systems used in law enforcement can be trained to make fair and unbiased decisions, reducing the risk of discriminatory practices.
Collaboration and Future Research
The development of FairDeDup was a collaborative effort involving multiple researchers. Eric Slyman worked closely with Stefan Lee, an assistant professor at the OSU College of Engineering, as well as Scott Cohen and Kushal Kafle from Adobe. This collaboration highlights the importance of interdisciplinary research in tackling complex challenges such as AI bias.
Moving forward, further research is needed to refine FairDeDup and explore its applications in various domains. The team plans to continue their work on improving the technique and investigating new ways to make AI training more fair and cost-effective.
Conclusion
The development of FairDeDup marks a significant advancement in the effort to create fair and unbiased AI systems. By addressing social biases in training datasets, this innovative technique paves the way for AI technologies that are more inclusive and equitable. As AI continues to play an increasingly important role in our lives, ensuring its fairness is crucial for building a just and equitable society.
Resarch Paperlink : https://openaccess.thecvf.com/content/CVPR2024/papers/Slyman_FairDeDup_Detecting_and_Mitigating_Vision-Language_Fairness_Disparities_in_Semantic_Dataset_CVPR_2024_paper.pdf