How Generative Ai Pipeline Work ?
Generative AI (GenAI) has revolutionized digital content creation, leveraging advanced language models (LM) from leaders like OpenAI and Google. By collecting data, vectorizing it with metadata, and using sophisticated embedding models, GenAI enhances text, image, and audio generation. This technology enables smarter, context-aware applications, transforming how we interact with AI-driven tools and paving the way for innovative solutions in various industries.
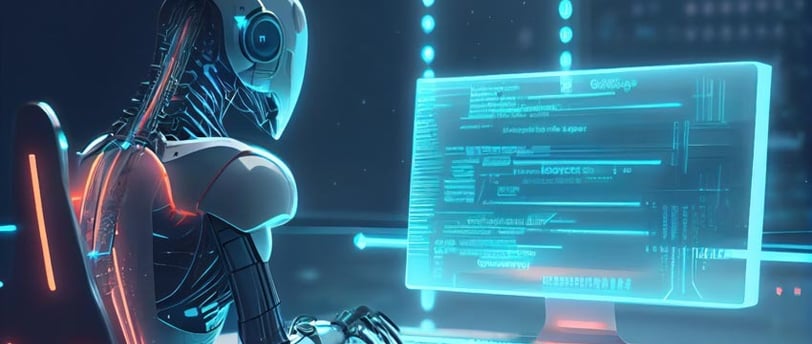
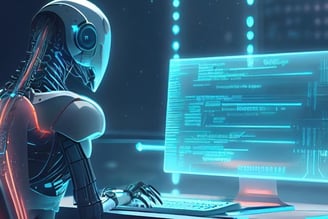
In recent years, artificial intelligence (AI) has achieved remarkable progress, overcoming previous limitations through advancements in algorithms, computing power, and data collection. A notable subset of AI, Generative AI (GenAI), has particularly flourished, capable of producing digital content such as text, images, and audio based on existing data. Among GenAI's innovations are language models (LM), sophisticated neural networks adept at interpreting, summarizing, and generating text. These models offer intelligent responses by understanding word relationships, with major contributions from industry leaders like OpenAI, Google, Microsoft, Hugging Face, Anthropic, and Mistral.
Data Collection
Data collection is a pivotal first step in harnessing information for diverse business objectives. When developing consumer-facing chatbots or other AI-driven applications, selecting relevant data sources is crucial. These sources might include company portals (e.g., SharePoint, Confluent, document storage) or internal APIs. Establishing a push mechanism is ideal to ensure timely updates of the Large Language Model (LLM) application for end consumers.
Vectorization or Embedding
Vectorization involves enriching data by incorporating metadata such as authorship, date, and contextual details. This integration enhances data retrieval, enabling smarter and more targeted searches. Metadata can be found within the portal or the document’s own metadata. For documents linked to business objects like cases, customers, or employee information, relevant data must be extracted from relational databases. Security concerns can be addressed by including security metadata at this stage, ensuring secure data access and aiding in subsequent retrieval stages.
Vector Representations
Converting text and images into vector representations is crucial, achieved using Large Language Model (LLM) embedding models. For documents, the process involves chunking the text followed by encoding, preferably with on-premises zero-shot embedding models. This comprehensive approach ensures effective vectorization and metadata integration, enhancing the overall data processing pipeline.
Vector Storing or Indexing
Vector indexing is essential for managing vector representations, commonly utilized in Large Language Model (LLM) systems. Vector databases or indexes are vital for efficiently storing and indexing these embeddings. Serving as the “LLM source of truth,” these databases must remain synchronized with the underlying data sources and documents. Real-time indexing is crucial for LLM applications catering to customers or generating business-related information, preventing inconsistencies between the LLM app and its data sources.
Information Retrieval
Extracting information through a Retrieval-Augmented Generation (RAG) approach involves obtaining pertinent context from a document. This method allows real-time responses to user queries by retrieving relevant data from an index and passing it to the model for processing.
Conclusion
Generative AI emphasizes prompt-based content generation using sophisticated language models. This shift transforms data processing approaches, showcasing Generative AI's versatility in producing more sophisticated and context-aware outputs. Generative AI, with its advanced capabilities and innovative methodologies, is redefining how we interact with and utilize digital content, providing a robust foundation for intelligent decision-making in various environments.