Google DeepMind AI Robot Set to Beat Humans at Table Tennis! 🏓🤖
Discover how Google DeepMind's AI-powered robot is mastering table tennis, blending advanced computer vision and machine learning to surpass human capabilities
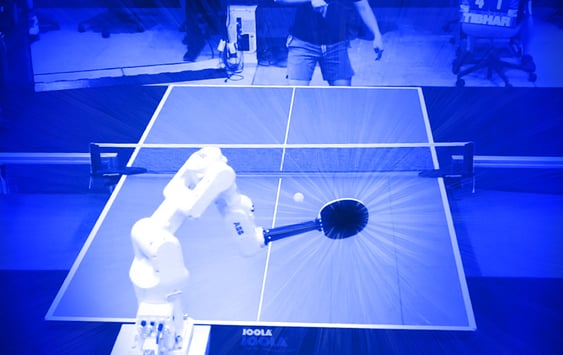
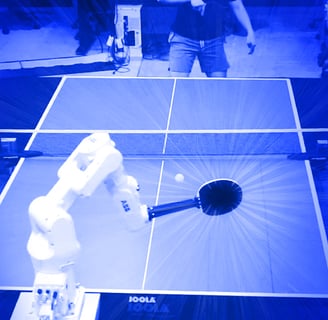
Artificial intelligence (AI) has rapidly advanced in recent years, pushing the boundaries of what machines can do. From mastering complex games like chess and Go to now challenging humans in physical activities like table tennis, AI is reshaping our understanding of technology's capabilities. Google DeepMind, a leader in AI research, has recently made headlines by developing a robot that can play table tennis at a human level. This achievement marks a significant milestone in the intersection of AI, robotics, and sports.
In this blog, we will explore the detailed architecture and modeling that went into developing this groundbreaking technology, the benefits and impacts of such advancements, and what we can expect in the next few years as AI continues to evolve. We will also discuess how AI is poised to transform competitive environments, how computer vision plays a crucial role in these developments.
What is Google’s DeepMind Table Tennis Player? 🤖
Google's DeepMind has developed an advanced table tennis-playing robot designed to compete at a human level, known as "Project Astra." 🤖 This robot represents a significant breakthrough in AI and robotics, showcasing DeepMind's ability to create a system that can not only play but also learn and adapt in real-time during table tennis matches.
The robot utilizes a sophisticated architecture combining hierarchical and modular policies. At its core, the system is built on low-level controllers responsible for executing specific skills, like returning a fastball or performing a spin shot. 🎾 These controllers are managed by a high-level AI that determines the best strategy during gameplay, allowing the robot to adjust to the opponent's style, whether they are a beginner or an advanced player.
One of the most impressive features of this robot is its ability to perform in zero-shot conditions, meaning it can adapt and perform well against opponents it has never encountered before without additional training. 🏆 In a series of matches against human players, the robot achieved a 45% win rate, including victories over intermediate-level human players.
DeepMind's table tennis robot is not just about winning games; it symbolizes the broader potential of AI in mastering complex, real-world tasks that require quick thinking, adaptability, and precision—skills that are crucial not only in sports but in various other domains as well.
This innovation also reflects Google's broader ambitions to enhance AI-driven search technologies, aiming to improve how information is retrieved and used across the internet. By pushing the boundaries of AI's capabilities in tasks like table tennis, DeepMind is setting the stage for future advancements in AI that could revolutionize everything from competitive sports to everyday applications. 🚀🤖
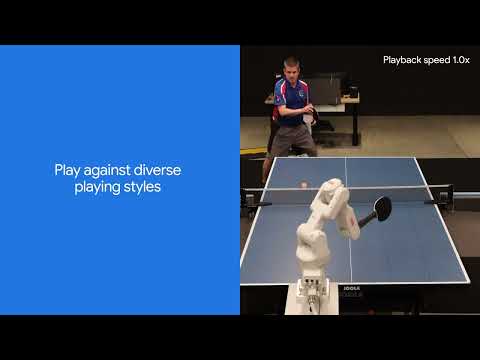
Google DeepMind Research Paper Review 📄🤖🔍
Robotics has made huge Development in Last Few Year 🤖, particularly in AI systems that perform complex tasks autonomously. A fascinating example is this research paper "Achieving Human-Level Competitive Robot Table Tennis." 🏓 It showcases a robot that competes with tennis players, demonstrating quick decision-making ⚡ and precise movements.🌟
The Challenge: Competing with Humans in Real-Time 🏓🤖
Developing a robot that can interact with and compete against humans in a dynamic and unpredictable environment like table tennis presents a unique challenge. Unlike other robotic tasks, table tennis requires real-time decision-making, rapid adaptation, and precise execution—all within milliseconds! ⏱️✨ The researchers aimed to overcome the limitations of previous systems that struggled to perform consistently in real-world settings, especially in tasks that demand such agility and accuracy. ⚡🤔
1. System Design and Hardware Integration 🖥️⚙️
The robot's design incorporates high-speed cameras, sensors, and actuators to facilitate real-time interaction with the game environment. The cameras track the ball’s trajectory 🎥, while the actuators control the paddle's movement with precision. This hardware setup is optimized for the speed and accuracy needed in table tennis, ensuring the robot can effectively respond to the fast-paced game.
2. Hierarchical Policy Architecture 🧠🔄
A significant innovation in this research is the hierarchical policy architecture, which breaks down the complex task into two primary levels:
Low-Level Controllers: These controllers execute specific table tennis skills, such as hitting, spinning, and positioning the paddle. Each low-level controller is a neural network trained to optimize different actions, enabling the robot to perform precise movements based on incoming sensory data. 🥇
High-Level Policy: Governed by a reinforcement learning framework, the high-level policy determines which low-level controller to activate at any given moment. This decision is based on the current state of the game, allowing the robot to adapt its strategy in real-time. The modularity of this architecture is crucial, as it helps the system handle the complexities of table tennis by breaking the task into more manageable components. ⚡📈
3. Training in Simulation: The Sim-to-Real Approach 🎮🌍
Training robotic systems in real-world environments can be impractical due to time, cost, and hardware wear. To address these challenges, the researchers employed a sim-to-real transfer approach, where the robot's skills were initially developed in a simulated environment using a physics engine that accurately mimics the dynamics of table tennis.
Domain Randomization: A key technique during simulation was domain randomization, where various simulation parameters (like ball speed, spin, and noise levels) were varied randomly. This approach helps the robot learn a robust policy that can generalize to the real world, preparing it for the variability and unpredictability of real-world scenarios. 🎲
Fine-Tuning with Real-World Data: After extensive training in simulation, the robot’s policies were fine-tuned using real-world data. This step is essential for adapting the learned policies to the nuances of the real environment, such as slight differences in ball behavior or lighting conditions.
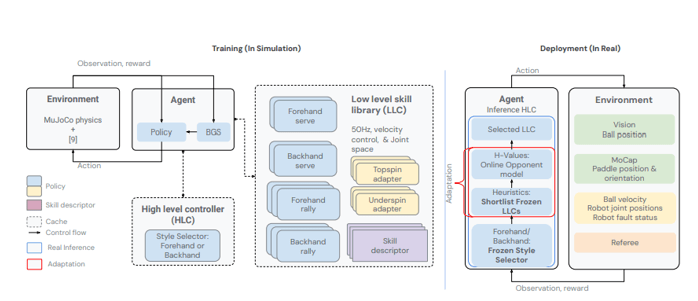
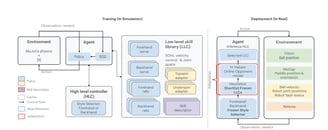
Physics-Based Simulation 🎮⚡
The researchers employed a sophisticated physics engine that replicates the dynamic nature of table tennis, creating a realistic training environment for the robot. This engine accurately simulates the ball's trajectory, spin, and bounce, enabling the robot to learn and adapt to various scenarios it might encounter during a real game. 🏓 This simulation is crucial because it allows the robot to experience a wide range of possible game states, from basic volleys to complex spins and fast-paced exchanges. 🎾
Sim-to-Real Transfer 🌍➡️🤖
One of the core challenges in robotics is the discrepancy between simulated and real-world environments. This paper tackles this issue by implementing a sim-to-real transfer learning approach. After extensive training in the simulated environment, the researchers fine-tuned the robot's policies with real-world data. 🔄 This step is essential for bridging the gap between simulation and actual gameplay, ensuring the robot's performance is robust and effective under real-world conditions. The fine-tuning process involves adjusting the robot's learned behaviors to account for real-world nuances, such as slight variations in ball behavior due to imperfections in the playing surface or environmental factors like lighting. 🌟
Reinforcement Learning for High-Level Policy 🎮🤖
The high-level policy was trained using reinforcement learning, where the robot received rewards for successful interactions, such as returning the ball or winning a point. 🏓🏆 This reinforcement learning framework enabled the robot to explore various strategies and optimize its gameplay over time. 🌟 By continuously learning from its experiences, the robot can adapt its approach and improve performance, ultimately enhancing its ability to compete effectively against human players. ⚡📈
Evaluation and Testing 📊🏆
The real-world evaluation phase involved rigorous testing through 29 matches against human opponents. These matches were designed to assess the robot's capabilities across various aspects, including reaction time, precision in returning the ball, and overall strategy. ⚡ The metrics recorded during these matches, such as win rates and return accuracy, provided valuable insights into the robot's strengths and areas for improvement. The researchers analyzed these metrics to evaluate the robot's performance and identify any gaps in its gameplay that could be addressed in future iterations. 🔍
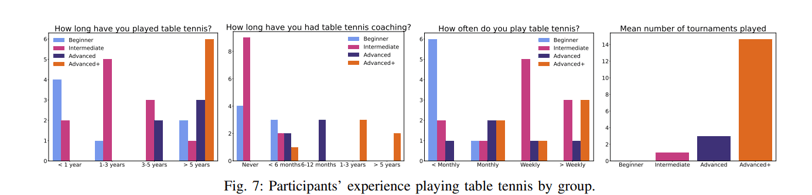
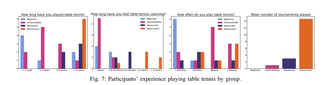
Challenges and Future Work 🚀🔧
While the robot’s performance is impressive, the research identified key challenges that need to be addressed. The transition from simulation to real-world conditions, although effective, isn’t flawless. Further refinement is required in the sim-to-real transfer process, potentially through more advanced simulation techniques or enhanced real-world data integration. Additionally, the robot’s strategic decision-making could benefit from incorporating more sophisticated machine learning methods, like deep reinforcement learning or hybrid models that blend rule-based systems with learning algorithms. 🧠💡
Conclusion
The research presented in “Achieving Human-Level Competitive Robot Table Tennis” marks a significant advancement in the field of robotics. By integrating hierarchical policy architecture, reinforcement learning, and sim-to-real transfer techniques, the researchers have developed a robotic system capable of competing with human players in one of the most dynamic and challenging environments. 🏓✨ This work not only pushes the boundaries of what is possible in robotics but also opens up new avenues for future research and applications across various fields. 🌍 The success of this project highlights the potential for robotics and traditional boundaries and operate effectively in complex, real-world scenarios. As researchers continue to refine these techniques, we can anticipate even more sophisticated robotic systems capable of performing tasks once considered the exclusive domain of humans. ⚡ Whether in sports, manufacturing, or healthcare, the future of robotics is undoubtedly exciting, and this research is a significant step toward realizing that future. 🚀🔍
Important Resources :
https://deepmind.google/research/publications/107741/
https://sites.google.com/view/competitive-robot-table-tennis
https://arxiv.org/pdf/2408.03906
🚀 Transform Your Business with XpandAI! 🌟
Ready to harness the power of AI?
XpandAI offers customized solutions to meet your unique needs! Whether it’s enhancing customer interactions, automating processes, or exploring new AI technologies, our expert team is here to help. 🤝✨
🌐 Visit agent.xpandai.com to discover how our AI Agent can revolutionize your operations!
📅 Book a consultation today and step into the future with intelligent, adaptable AI Solutions! 💼💡