Google AMIE: When AI Learns to ‘See’ in Medical Diagnostics
Digital heart made up of binary as Google gives its diagnostic AI the ability to understand visual medical information with its latest research on AMIE (Articulate Medical Intelligence Explorer) that promises to one day assist clinicians and patients and deliver powerful healthcare advancements.
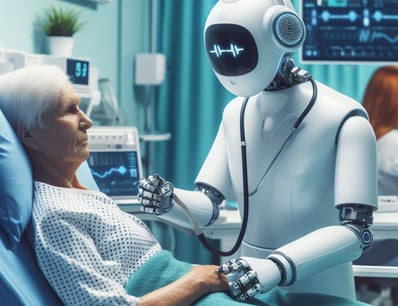
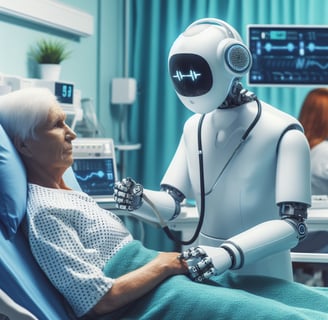
Imagine chatting with an AI about a health concern—not only processing your words, but also examining a photo of that worrying rash or interpreting your ECG printout. Google’s latest research on AMIE (Articulate Medical Intelligence Explorer) makes this vision a reality, equipping its diagnostic AI with “vision” to understand and reason about medical images. Building on earlier text-based successes published in Nature, AMIE aims to bridge the gap between conventional chatbots and human clinicians.
The Case for Multimodal AI in Medicine
Visual Clues Drive Diagnoses
Clinicians routinely rely on visual evidence—skin lesions, imaging studies, vital-sign graphs—to guide their decision-making. Text-only systems simply can’t tap into this critical dimension.Rich Conversations Require Rich Data
Modern messaging apps allow users to share images and documents seamlessly. Yet most AI agents ignore these multimodal inputs, limiting their usefulness in telemedicine and remote triage.Key Research Question
“Can large language models conduct diagnostic clinical conversations that incorporate complex multimodal information?”
Google’s team set out to answer this by teaching an AI not just to chat, but to look and reason.
How AMIE Works
1. Gemini 2.0 Flash at the Core
Advanced LLM Backbone: AMIE is powered by Google’s Gemini 2.0 Flash, renowned for its language understanding and generation.
State-Aware Reasoning: Rather than following a linear script, AMIE maintains an internal “state” tracking what it knows, what remains uncertain, and which visual inputs—like a rash photo or lab report—it needs next.
2. A High-Fidelity Simulation Lab
To train and evaluate AMIE safely, Google created a lifelike virtual clinic:
Curated Medical Images:
Dermatology: SCIN dataset
ECG Tracings: PTB-XL database
Synthetic Patient Histories: Generated by Gemini to add realistic context.
Automated Scoring: Scripts measured diagnostic accuracy, hallucination rates, and management plan quality.
OSCE-Style Evaluation: AI vs. Human Clinicians
Objective Structured Clinical Examination (OSCE) is how medical students are tested. Google mirrored this in a controlled study:
Scenarios: 105 standardized cases acted by trained performers.
Arms:
AMIE Chat: Patients uploaded images/documents.
Human PCPs: Conducted identical interactions.
Review Panel:
Specialists (dermatology, cardiology, internal medicine)
Patient Actors assessing communication and empathy
Key Findings
Superior Image Interpretation: AMIE outperformed PCPs in reading dermatology photos and ECG tracings.
Higher Diagnostic Accuracy: Experts rated AMIE’s differential diagnoses as more accurate and complete.
Enhanced Empathy: Patient actors found the AI more empathetic and trustworthy in text exchanges.
Safety Parity: AMIE’s rate of image-based errors matched human clinicians—no significant increase in hallucinations.
Early Insights with Gemini 2.5 Flash
In preliminary tests swapping in Gemini 2.5 Flash, AMIE showed further gains in top-3 diagnostic accuracy and management quality. However, Google underscores that expert physician review remains essential to validate these improvements.
Limitations & the Road Ahead
Simulation vs. Reality: Actor-driven OSCEs lack the full complexity of real patient encounters—nonverbal cues, unpredictable behaviors, and emotional nuance.
Static Media Only: Current tests use still images and documents; real telehealth involves live video and audio.
Clinical Trials: Google has partnered with Beth Israel Deaconess Medical Center to pilot AMIE under ethical oversight and patient consent.
Future development will focus on integrating with electronic health records, handling real-time video/audio, and rigorous safety monitoring.
Implications for Healthcare
Enhanced Telemedicine: Patients can share clinical images seamlessly during virtual consults.
Efficient Triage: Automated pre-visit assessments could flag urgent cases more quickly.
Decision Support: Clinicians gain a reliable second opinion on image interpretation.
Patient Empowerment: Conversational AI that “sees” helps patients understand their own health data.
Conclusion
Google’s AMIE marks a pivotal step toward truly multimodal medical AI—one that not only chats, but also sees and reasons with the same visual cues human clinicians do. While still in research and simulation, AMIE’s OSCE-style performance is both promising and provocative. As it advances into real-world trials, AMIE holds the potential to revolutionize telehealth, emergency triage, and decision support—augmenting clinicians and empowering patients alike.
Ready to explore how AI can transform your healthcare solutions?
Book A Call With Us learn how our team builds tailored multimodal AI agents that see, understand, and assist.