Enhancing Computational Imaging: Deep Learning with Limited Data
Discover groundbreaking methods reducing errors in computational imaging using deep learning with limited data. Explore advancements presented at ICML 2024
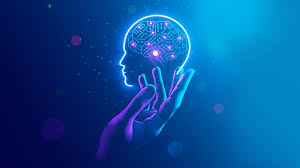
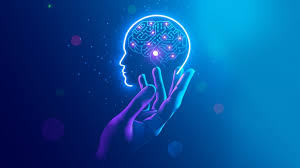
In recent years, deep learning has significantly advanced computational imaging, a field integral to various applications such as medical diagnostics, remote sensing, and scientific research. However, these advances often require extensive datasets, which are not always feasible. Two pivotal studies—one on training deep learning models with limited data to reduce errors in computational imaging and another on addressing prior mismatch in PnP-ADMM with nonconvex convergence analysis—present promising solutions.
Deep Learning with Limited Data
Key Challenges and Innovations
Training with Limited Data
Deep learning models typically require large datasets to achieve high accuracy. However, acquiring such datasets can be challenging, particularly in specialized fields like medical imaging. Researchers have developed innovative methods to train these models with limited data, significantly reducing computational errors.
New Methodologies
A novel approach to training deep learning models with limited data. This method could transform how computational imaging is performed, making it more accessible and efficient.
Practical Implications
Reducing the amount of data needed for training can lower costs and speed up the development process, enabling faster deployment of advanced imaging solutions in critical areas like healthcare.
PnP-ADMM and Prior Mismatch

Understanding PnP-ADMM
Plug-and-Play (PnP) priors integrate physical measurement models with image priors specified using image denoisers. Despite their success, issues arise due to distribution mismatch between training and testing data, which the ICML 2024 poster addresses.
Addressing Prior Mismatch
The study presents new theoretical and numerical results on prior distribution mismatch and domain adaptation in PnP-ADMM. It provides an explicit error bound for the mismatch, contributing significantly to the existing literature.
Enhancing Performance
Through robust analysis, the study suggests strategies to close performance gaps caused by mismatched denoisers, showcasing the robustness of PnP-ADMM to prior distribution mismatch.
Collaborative Efforts
Both studies underline the importance of bridging theoretical advancements with practical applications. Collaboration among researchers, industry practitioners, and policymakers is crucial for translating these innovations into real-world solutions.
Future Directions
Future research should focus on refining these methods, exploring their applications in diverse fields, and ensuring their accessibility to a broader audience.
Conclusion
The advancements in deep learning with limited data and addressing prior mismatch in PnP-ADMM mark significant milestones in computational imaging. These innovations promise to enhance the accuracy and efficiency of imaging techniques, with far-reaching implications across various domains. As research progresses, we can anticipate more groundbreaking developments, further solidifying the role of deep learning in transforming computational imaging.
Resarch Paper Link : https://proceedings.mlr.press/v235/shoushtari24a.html
Github Link : https://github.com/wustl-cig/MMPnPADMM