Detailed Review: How TX-LLM, Fine-Tuned from PaLM 2, Predicts Key Properties for Therapeutic Development
Discover how TX-LLM, a large language model fine-tuned from PaLM 2, predicts properties of entities crucial for therapeutic development, advancing the field of medicine
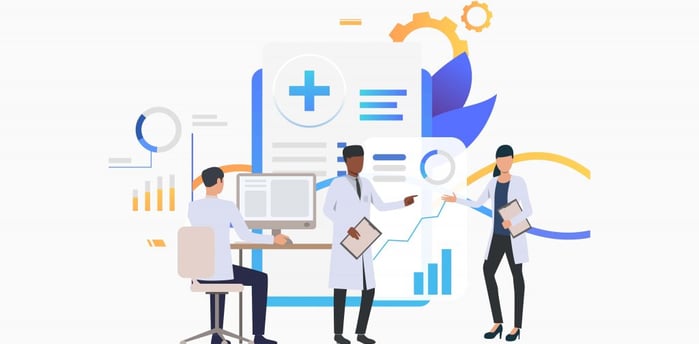
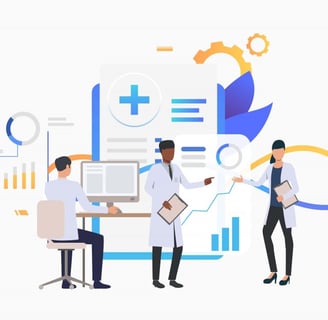
Artificial Intelligence (AI) has become a cornerstone in various sectors, but one of its most promising applications is in healthcare, especially therapeutic development. One recent breakthrough is TX-LLM, a large language model (LLM) fine-tuned from PaLM 2, that has the unique ability to predict properties of many entities relevant to therapeutic development. With this advanced AI model, researchers and healthcare professionals can now access more accurate predictions, improving the efficiency and effectiveness of developing new treatments and drugs.
What is TX-LLM?
TX-LLM is a fine-tuned version of PaLM 2, an advanced language model developed by Google. PaLM 2 is part of the Pathways Language Model (PaLM) family, designed for versatility in natural language processing (NLP) tasks. TX-LLM, however, is specifically trained to understand and predict properties of biological entities, chemical compounds, and other critical components of therapeutic development.
The beauty of TX-LLM lies in its specialization. While general-purpose LLMs are great for tasks like answering questions or summarizing text, TX-LLM’s unique fine-tuning enables it to predict the properties of molecules, proteins, and other substances that play a pivotal role in creating new therapies and drugs.
How Does TX-LLM Work?
TX-LLM employs machine learning techniques to analyze massive datasets of biological and chemical information. The model has been trained on a wide array of scientific literature, research papers, and datasets that focus on therapeutic compounds, drug interactions, and molecular properties. Here's a breakdown of how it functions:
Data Collection and Preprocessing: Large datasets of chemical compounds, biological entities, and molecular interactions are fed into the model. These datasets undergo extensive preprocessing to remove noise and ensure that only the most relevant information is retained.
Fine-Tuning on PaLM 2: TX-LLM is a refined version of PaLM 2, but with additional training data focused on therapeutic development. Fine-tuning allows the model to specialize in this niche, gaining a deeper understanding of the biochemical and pharmacological domains.
Property Prediction: The model predicts the properties of various entities—such as the solubility of a compound, the potential effectiveness of a drug against a particular disease, or the likely side effects of a combination of drugs. It makes these predictions based on its deep learning architecture, which analyzes relationships between entities within the data.
Continuous Learning: TX-LLM evolves as it is exposed to new data, making it an adaptable tool for scientists and researchers. Its predictions become more accurate over time as more data about new therapeutic discoveries is fed into the system.
Key Applications of TX-LLM in Therapeutic Development
1. Drug Discovery
One of the most promising applications of TX-LLM is in drug discovery. Developing new drugs is traditionally a long, complex, and expensive process. TX-LLM can dramatically speed up this process by predicting which compounds are most likely to be effective against specific diseases. This means fewer trial-and-error experiments and more targeted, efficient research.
For instance, TX-LLM can predict how a potential drug might interact with a specific protein in the body, identifying whether the compound has the desired therapeutic effect or if it might cause harmful side effects. By predicting these interactions early on, researchers can focus on the most promising candidates, reducing the time and cost of drug development.
2. Molecular Property Analysis
TX-LLM can analyze the properties of molecules and predict their behavior in biological systems. This is particularly useful for understanding how certain compounds can be used in therapies. For example, the model can predict whether a molecule is likely to be absorbed efficiently by the human body or if it will be broken down too quickly to be effective.
3. Personalized Medicine
TX-LLM can also contribute to the development of personalized medicine, where treatments are tailored to individual patients based on their genetic makeup, lifestyle, and specific health conditions. By analyzing data from individual patients, TX-LLM can help doctors predict which drugs will be most effective for each person, minimizing the risk of adverse reactions and improving outcomes.
4. Repurposing Existing Drugs
Another fascinating use case for TX-LLM is in drug repurposing. By analyzing existing data on approved drugs, TX-LLM can predict whether these drugs might be effective in treating other diseases. This approach saves significant time and resources compared to developing a brand-new drug from scratch.
Advantages of TX-LLM Over Traditional Models
While traditional models and methods for drug discovery and therapeutic development rely on years of experimental data and slow trial processes, TX-LLM offers a faster, more data-driven approach. Here are some key advantages:
Speed: TX-LLM can analyze vast datasets and make predictions in a fraction of the time it would take for human researchers to do the same manually.
Accuracy: The model's fine-tuning on therapeutic datasets means it makes highly accurate predictions about molecular and biological properties, reducing the likelihood of failure in later stages of drug development.
Cost-Effectiveness: By streamlining the discovery process and predicting outcomes earlier, TX-LLM can save billions of dollars in drug development costs.
Scalability: Traditional research teams can only work on a limited number of projects at a time. TX-LLM, on the other hand, can analyze multiple compounds or potential therapies simultaneously.
TX-LLM represents a significant step forward in the world of therapeutic development. Its ability to predict molecular properties and interactions allows researchers to focus their efforts on the most promising treatments, accelerating the path to clinical trials and eventual approval.
As this technology evolves, we can expect even more impressive applications, including the potential to cure complex diseases or develop entirely new types of treatments. TX-LLM's influence could extend beyond drug discovery, impacting areas like vaccine development, gene therapy, and even preventive medicine.
Conclusion
TX-LLM isn't just another large language model—it's a groundbreaking tool that has the potential to revolutionize therapeutic development. By leveraging the power of AI and fine-tuning it for a specific purpose, researchers now have the ability to make faster, more accurate predictions, reducing costs and time in the process. As AI continues to advance, TX-LLM will undoubtedly play a key role in shaping the future of medicine, bringing us closer to more effective, personalized, and accessible treatments.
Resources : https://research.google/blog/tx-llm-supporting-therapeutic-development-with-large-language-models/
https://arxiv.org/pdf/2406.06316
Boost your business with XpndAI's LLM-powered solutions! From smart chatbots to AI-driven insights, we help automate and grow your business efficiently. Book A Call Below !