Detail Review of OpenAI o3-mini System Card
Explore OpenAI o3-mini, a cost-efficient, high-performance reasoning model optimized for STEM tasks. Discover its features, pricing & future AI innovations today.
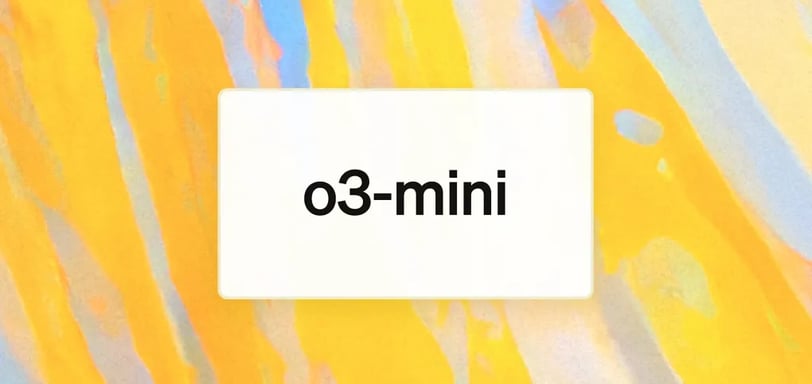
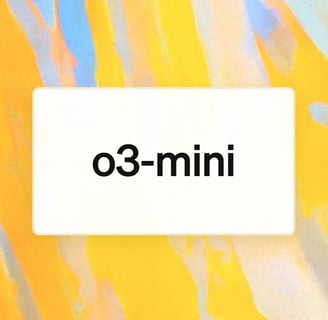
OpenAI’s latest release, o3‑mini, is redefining cost‑effective reasoning by delivering exceptional STEM capabilities while keeping operational costs low. In this post, we explore the inner workings of the o3‑mini system card, examine its benefits and limitations, and compare its performance and cost efficiency with previous models such as o1‑mini and GPT‑4o because we know that in the current situation there is no comparison between deepseekR1 and openai o3-mini.
The Evolution: From o1‑mini to o3‑mini
OpenAI has been on a continuous quest to balance efficiency with robust reasoning abilities. The o3‑mini represents a significant leap forward in this journey. Here’s how it stands out from its predecessor:
Enhanced STEM Capabilities:
The model is optimized for math, coding, and science. With adjustable reasoning effort settings (low, medium, and high), developers can fine‑tune the balance between speed and analytical depth. In benchmark evaluations—such as the AIME competition and PhD‑level science tests—o3‑mini not only matches but often outperforms the broader o1 model while delivering faster responses.Developer‑Friendly Features:
o3‑mini introduces several critical upgrades including:Function Calling: Enables seamless integration with external tools.
Structured Outputs: Supports JSON Schema constraints for well‑defined automated workflows.
Developer Messages: A new message type replacing traditional system messages for more flexible instruction handling.
These enhancements make it significantly easier for developers to integrate the model into production systems.
Cost and Latency Improvements:
The model offers a 95% reduction in per‑token pricing compared to earlier GPT‑4 pricing models and boasts a 2,500ms faster time-to-first-token than the o1‑mini. This makes o3‑mini an attractive option for applications that require high throughput with minimal latency.
How Does o3‑mini Work?
At its core, o3‑mini is engineered to deliver precise, rapid reasoning with built‑in flexibility:
Adjustable Reasoning Effort
Low and Medium: Ideal for routine queries where speed is critical.
High: Activates deeper, more complex analysis for challenging tasks like advanced coding problems or complex scientific reasoning.
This multi‑tier approach allows users to choose the right balance between response speed and analytical depth based on their specific needs.
Safety and Alignment
A standout feature of o3‑mini is its use of deliberative alignment. The model is trained to internally evaluate human‑written safety specifications before responding, significantly reducing major errors and providing robust safeguards against misuse—even outperforming GPT‑4o on safety benchmarks.
Integration with Real‑Time Tools
The integration with search capabilities means o3‑mini can now retrieve up‑to‑date information from the web and include precise citations in its outputs. This step toward dynamic, verifiable responses further enhances its utility in real‑world applications.
Benefits of Using o3‑mini
The design of o3‑mini brings several notable advantages:
1. Cost‑Effective Intelligence
Lower Operational Costs: With dramatically reduced per‑token pricing, o3‑mini makes high‑quality reasoning accessible to a broader range of users—from individual developers to large enterprises.
2. Optimized for STEM and Coding
Enhanced Performance: Whether it’s competitive math challenges or advanced coding tasks, the model’s specialized optimizations ensure higher accuracy and speed compared to its predecessors.
3. Developer-Centric Features
Ease of Integration: With support for function calling, structured outputs, and developer messages, integrating o3‑mini into existing workflows is simpler and more efficient.
4. Reduced Latency
Faster Response Times: With a significantly lower latency than previous models, o3‑mini is well‑suited for real‑time applications where every millisecond counts.
Limitations and Areas for Improvement
Despite its many strengths, o3‑mini has a few limitations:
Lack of Vision Support:
Unlike some of the multimodal models in the OpenAI portfolio, o3‑mini is strictly text‑based. Developers needing visual reasoning capabilities must continue using models like OpenAI o1.Occasional Hallucinations and Formatting Errors:
While the model is robust, it can sometimes generate minor inaccuracies or formatting issues. These challenges are expected to improve over time as the model is further refined.Trade‑Offs Between Cost and Precision:
The adjustable reasoning settings allow for cost savings at lower effort levels; however, this may come at the expense of precision in more complex tasks. Users must carefully select the appropriate mode based on their requirements.
Cost Comparison: o3‑mini, o1‑mini, and GPT‑4o
When evaluating models for both performance and cost, o3‑mini stands out:
o3‑mini vs. o1‑mini:
With a tripling of rate limits for certain users (from 50 to 150 messages per day for Plus and Team users) and lower latency, o3‑mini provides a superior cost‑to‑performance ratio while matching or exceeding o1‑mini in technical benchmarks.o3‑mini vs. GPT‑4o:
Although GPT‑4o offers robust multimodal capabilities, it comes with higher operational costs. o3‑mini, with its leaner pricing and optimized reasoning for STEM tasks, offers an attractive alternative for users who do not require advanced visual processing.Enterprise Advantages:
The model’s enhanced developer features and integration into platforms like Microsoft Azure OpenAI Service expand its appeal for enterprise applications that demand efficiency, scalability, and reduced costs.
Looking to the Future: Deep Research Integration
The advancements in o3‑mini set the stage for future innovations. OpenAI’s upcoming Deep Research feature—powered by a version of the next‑generation o3 model—is designed to automate complex, multi‑step research tasks. As detailed by Fone Arena, Deep Research can autonomously analyze and synthesize vast online sources, delivering detailed, research‑grade reports in minutes.
This evolution not only underscores the potential for automated research but also hints at the future integration of real‑world actions and enhanced tool support in AI models.
Conclusion
OpenAI o3‑mini is a landmark in the evolution of cost‑effective AI reasoning but still not matched with deep seek R1 . By delivering specialized STEM optimizations, flexible reasoning effort settings, and enhanced developer features—all while significantly reducing costs—o3‑mini paves the way for scalable, high‑performance AI applications. Although there are areas for improvement, especially in multimodal support and minor accuracy issues, the model’s design sets a promising foundation for the future of AI research and enterprise applications.
As OpenAI continues to innovate, the advancements seen in o3‑mini will undoubtedly influence the next generation of models, making high‑quality AI more accessible and efficient for all users.
Transform your business with XPNDAI AI Solutions! Automate customer interactions, cut costs, and boost efficiency with our affordable, cutting-edge AI tools. Get started today & Book A Call with us.