AI Revolutionizes Material Science with New Fingerprinting Technique
Discover how scientists are using AI and X-ray science to create material 'fingerprints,' offering unprecedented insights into material dynamics and behavior.
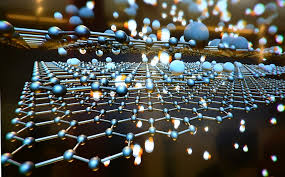
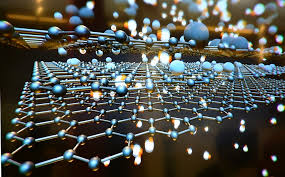
Researchers at the DOE/Argonne National Laboratory have unveiled a novel technique that leverages artificial intelligence (AI) and X-ray photon correlation spectroscopy (XPCS) to create "fingerprints" of materials. This innovative approach enables scientists to gain unprecedented insights into how materials change over time, especially under stress and relaxation conditions. The technique utilizes unsupervised machine learning algorithms to recognize hidden patterns in the arrangements of X-rays scattered by colloids, providing a deeper understanding of material dynamics.
Understanding Material Dynamics
Materials, much like living organisms, evolve and change over time. Their behavior under different conditions, such as stress and relaxation, is crucial for various applications in material science and engineering. Traditional methods of studying these changes involve collecting vast amounts of X-ray scattering data, which can be extremely complex and challenging to interpret without advanced computational tools.
X-ray Photon Correlation Spectroscopy (XPCS)
XPCS is a powerful technique used to study the dynamics of materials at the nanoscale. By analyzing the patterns of X-rays scattered by a sample, scientists can gain insights into the structural changes occurring within the material. However, the patterns generated by XPCS are often too intricate for manual analysis, necessitating the use of AI to decipher the data effectively.
Artificial Intelligence and Machine Learning
The new technique developed by Argonne researchers pairs XPCS with an unsupervised machine learning algorithm, a type of neural network that does not require expert training. This algorithm can autonomously learn to recognize patterns within the X-ray scattering data, transforming the complex patterns into simplified "fingerprints" of the materials.
Creating Material Fingerprints
The project, named Artificial Intelligence for Non-Equilibrium Relaxation Dynamics (AI-NERD), utilizes a specific type of neural network known as an autoencoder. Autoencoders are designed to compress data into a latent representation, or fingerprint, which retains only the essential information about the original image. This process allows the neural network to categorize and cluster similar fingerprints, creating a comprehensive map of the material's structural characteristics.
The Role of Neural Networks
Neural networks, inspired by the human brain, are adept at recognizing patterns in complex datasets. In the context of the AI-NERD project, the neural network analyzes the X-ray scattering patterns as regular images, identifying repeating patterns and categorizing them accordingly. This capability is crucial for understanding the intricate details of how materials evolve over time.
Advancing Material Science
The ability to create material fingerprints has far-reaching implications for material science. By providing a detailed map of how materials change under different conditions, this technique can aid in the development of new materials with tailored properties. For instance, understanding the stress and relaxation dynamics of polymers can lead to the creation of more durable and resilient materials for various industrial applications.
Enhanced Research Capabilities
The upgraded Advanced Photon Source (APS) facility at Argonne, set to generate X-ray beams 500 times brighter than its predecessor, will greatly benefit from the integration of AI techniques like AI-NERD. The enhanced brightness will produce even more complex datasets, which AI can efficiently analyze, furthering our understanding of material dynamics at an unprecedented scale.
Cross-disciplinary Collaboration
The success of the AI-NERD project highlights the importance of collaboration across different scientific disciplines. Researchers from Argonne's Advanced Photon Source, Center for Nanoscale Materials, and X-ray Science division worked together to develop and implement this technique. Such interdisciplinary efforts are crucial for tackling complex scientific challenges and driving innovation.
Conclusion
The development of a new AI-powered technique to create material fingerprints marks a significant advancement in the field of material science. By combining XPCS with machine learning, researchers can now gain deeper insights into the structural dynamics of materials, paving the way for new discoveries and applications. As the upgraded APS facility comes online, the integration of AI will be essential for managing and interpreting the vast amounts of data generated, ensuring that scientists can continue to push the boundaries of material science.